반응형
04-2 강의에서는 앞서 배운 내용을 가지고 프로그램을 돌려보는 것에 대해 국한되지 않고 기본적인 파이썬에서 코드에 대해서도 같이 정리할 예정이다.
csv 파일 읽기
'data-01-test-score.csv'라는 파일을 읽고자 한다. 내용은 다음과 같다.
#EXAM1, EXAM2, EXAM3, FINAL 73, 80, 75, 152 93, 88, 93, 185 89, 91, 90, 180 ... 76, 83, 71, 196 96, 93, 95, 142 |
파일을 읽기 위해서는 numpy를 import한다.
import numpy as np
loadtxt를 사용한다. load할 파일명과 seperate할 조건(,)과 데이터타입(float32)을 설정한다.
xy = np.loadtxt('data-01-test-score.csv', delimiter=',', dtype=np.float32)
Slicing
nums = range(5) # range is a built-in function that creates a list of integers
print nums # Prints "[0, 1, 2, 3, 4]"
print nums[2:4] # Get a slice from index 2 to 4 (exclusive); prints "[2, 3]"
print nums[2:] # Get a slice from index 2 to the end; prints "[2, 3, 4]"
print nums[:2] # Get a slice from the start to index 2 (exclusive); prints "[0, 1]"
print nums[:] # Get a slice of the whole list; prints "[0, 1, 2, 3, 4]"
print nums[:-1] # Slice indices can be negative; prints "[0, 1, 2, 3]"
num[2:4] = [8:9] # Assign a new sublist to a slice
print nums # Prints "[0, 1, 8, 9, 4]"
Indexing, Slicing, Iterating
a = np.array([1, 2, 3, 4, 5])
#array([1, 2, 3, 4, 5])
a[1:3]
#array([2, 3])
a[-1]
#5
a[0:2] = 9
a
#array[9, 9, 3, 4, 5]
b = np.array([[1, 2, 3, 4], [5, 6, 7, 8], [9, 10, 11, 12]])
# array([[1, 2, 3, 4],
# [5, 6, 7, 8],
# [9, 10, 11, 12]])
b[:,-1]
# array([2, 6, 10])
b[-1]
# array([9, 10, 11, 12])
b[-1, :]
# array([9, 10, 11, 12])
b[0:2, :]
# array([[1, 2, 3, 4],
# [5, 6, 7, 8]])
lab-04-3-file_input_linear_regression.py
# Lab 4 Multi-variable linear regression
import tensorflow as tf
import numpy as np
tf.set_random_seed(777) # for reproducibility
xy = np.loadtxt('data-01-test-score.csv', delimiter=',', dtype=np.float32)
x_data = xy[:, 0:-1]
y_data = xy[:, [-1]]
# Make sure the shape and data are OK
print(x_data, "\nx_data shape:", x_data.shape)
print(y_data, "\ny_data shape:", y_data.shape)
# data output
'''
[[ 73. 80. 75.]
[ 93. 88. 93.]
...
[ 76. 83. 71.]
[ 96. 93. 95.]]
x_data shape: (25, 3)
[[152.]
[185.]
...
[149.]
[192.]]
y_data shape: (25, 1)
'''
# placeholders for a tensor that will be always fed.
X = tf.placeholder(tf.float32, shape=[None, 3])
Y = tf.placeholder(tf.float32, shape=[None, 1])
W = tf.Variable(tf.random_normal([3, 1]), name='weight')
b = tf.Variable(tf.random_normal([1]), name='bias')
# Hypothesis
hypothesis = tf.matmul(X, W) + b
# Simplified cost/loss function
cost = tf.reduce_mean(tf.square(hypothesis - Y))
# Minimize
optimizer = tf.train.GradientDescentOptimizer(learning_rate=1e-5)
train = optimizer.minimize(cost)
# Launch the graph in a session.
sess = tf.Session()
# Initializes global variables in the graph.
sess.run(tf.global_variables_initializer())
for step in range(2001):
cost_val, hy_val, _ = sess.run([cost, hypothesis, train],
feed_dict={X: x_data, Y: y_data})
if step % 10 == 0:
print(step, "Cost:", cost_val, "\nPrediction:\n", hy_val)
Result
# train output
'''
0 Cost: 21027.0
Prediction:
[[22.048063 ]
[21.619772 ]
...
[31.36112 ]
[24.986364 ]]
10 Cost: 95.976326
Prediction:
[[157.11063 ]
[183.99283 ]
...
[167.48862 ]
[193.25117 ]]
1990 Cost: 24.863274
Prediction:
[[154.4393 ]
[185.5584 ]
...
[158.27443 ]
[192.79778 ]]
2000 Cost: 24.722485
Prediction:
[[154.42894 ]
[185.5586 ]
...
[158.24257 ]
[192.79166 ]]
'''
Queue Runners
파일이 큰 경우 메모리를 한번에 올리기에 힘든 경우 numpy를 쓰면 메모리가 부족하다고 나온다. 이런 경우를 대비해서 tensorflow에서는 Queue Runners라는 시스템이 만들어져 있다.
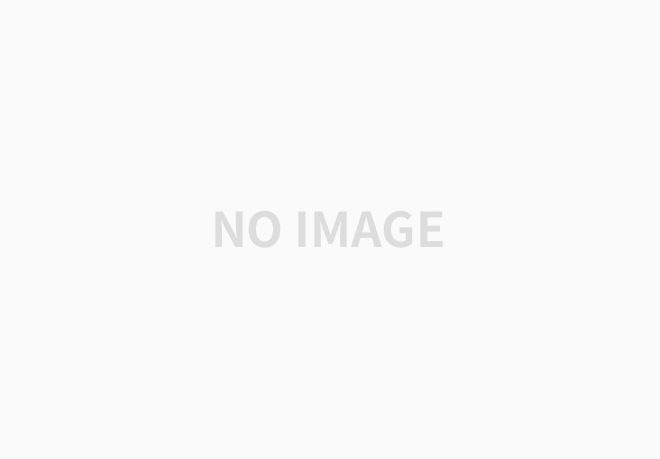
A, B, C 여러개의 파일을 읽어와서 queue에다가 쌓게된다. 그런다음 Reader로 연결해서 데이터를 읽은 다음 양식에 맞게 decoder를 해서 다시 큐에다 쌓는다.
#1
filename_queue = tf.train.string_input_producer(
['data-01-test-score.csv'], shuffle=False, name='filename_queue')
#2
reader = tf.TextLineReader()
key, value = reader.read(filename_queue)
#3
record_defaults = [[0.], [0.], [0.], [0.]]
xy = tf.decode_csv(value, record_defaults=record_defaults)
tf.train.batch
# collect batches of csv in
train_x_batch, train_y_batch = \
tf.train.batch([xy[0:-1], xy[-1:]], batch_size=10)
sess = tf.Session()
...
# Start populating the filename queue.
coord = tf.train.Coordinator()
threads = tf.train.start_queue_runners(sess=sess, coord=coord)
for step in range(2001):
x_batch, y_batch = sess.run([train_x_batch, train_y_batch])
...
coord.request_stop()
coord.join(threads)
lab-04-4-tf_reader_linear_regression.py
반응형
'Deep Learning lecture' 카테고리의 다른 글
ML lec 5-2 Logistic Regression의 cost 함수 설명 (0) | 2020.04.28 |
---|---|
ML lec 5-1: Logistic Classification의 가설 함수 정의 (0) | 2020.04.28 |
ML lab 04-1: multi-variable linear regression을 TensorFlow에서 구현하기 (new) (0) | 2020.04.27 |
ML lec 04 - multi-variable linear regression (*new) (0) | 2020.04.27 |
ML lab 03 - Linear Regression 의 cost 최소화의 TensorFlow 구현 (new) (0) | 2020.04.27 |
댓글